Transparency in AI: What Are The Legal Requirements for Informing Data Subjects About Automated Decisions
- Robert Westmacott
- Mar 17
- 6 min read
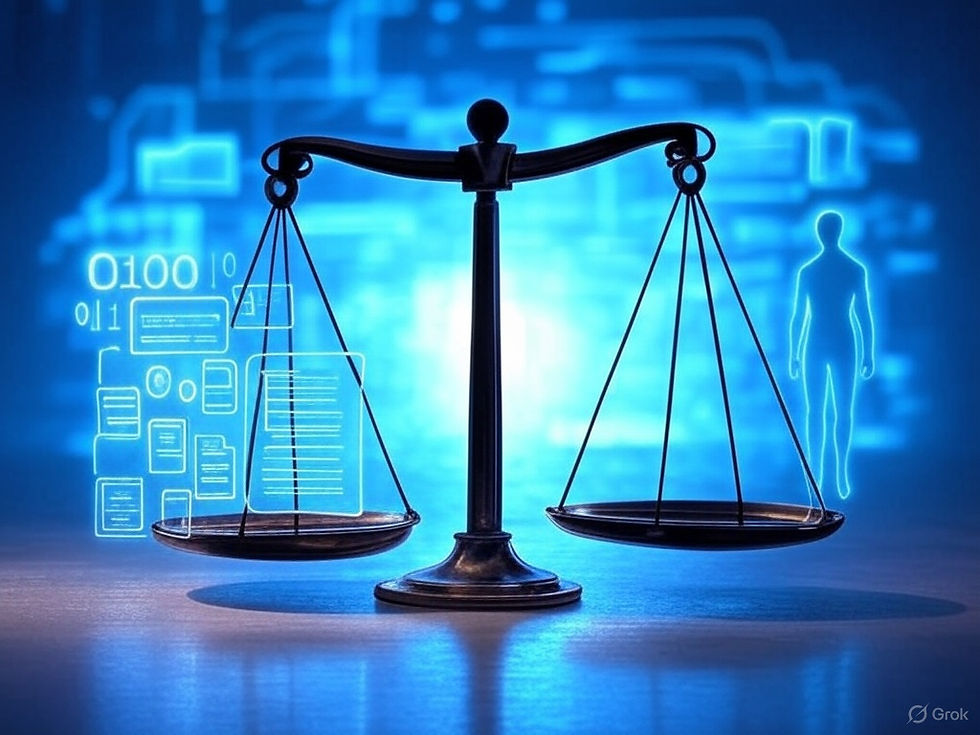
Introduction
Automated decision-making (ADM) has become a cornerstone of modern technology, leveraging artificial intelligence (AI), machine learning, and profiling to process vast amounts of data at unprecedented speeds.
From credit scoring to hiring algorithms, ADM systems are embedded in everyday decisions that significantly impact individuals data subjects in legal terms. These systems analyze personal data to make predictions or decisions without human intervention, often in areas like loan approvals, job candidate selection, or even healthcare diagnostics. While ADM offers organizations efficiency and scalability, it raises critical questions about transparency and fairness for individuals whose lives are affected by these decisions.
Transparency in ADM is vital because it empowers data subjects to understand how decisions affecting them are made, ensuring accountability and protecting their rights. Without clear information, individuals may be unaware of the processes shaping their opportunities, potentially leading to unfair outcomes or discrimination. However, providing this transparency often clashes with organisational interests, such as protecting proprietary algorithms or maintaining operational efficiency.
This blog post explores how much information must be provided to data subjects about ADM, delving into legal obligations, practical challenges, and best practices while maintaining an impartial perspective on this evolving issue.
Legal Frameworks
The General Data Protection Regulation (GDPR), enacted in the European Union in 2018, serves as the primary legal framework governing transparency in ADM. Articles 13, 14, and 15 of the GDPR mandate that data controllers inform data subjects about the existence of automated decision-making, including profiling, and provide "meaningful information about the logic involved" as well as the "significance and envisaged consequences" of such processing.
Article 22 further establishes a right for individuals not to be subject to decisions based solely on automated processing that produce legal or similarly significant effects, unless certain conditions are met (e.g., explicit consent or necessity for a contract). In such cases, data subjects must be granted the right to human intervention, to express their views, or to contest the decision.
The GDPR’s requirements have sparked debates, particularly around the so-called "right to explanation." Recital 71 suggests that individuals should receive an explanation of automated decisions, but scholars and regulators remain divided on whether this constitutes a legally binding right. The European Data Protection Board (EDPB) has issued guidelines emphasising that transparency must be meaningful, but the exact scope remains subject to interpretation, as seen in ongoing discussions as of early 2025.
Globally, other frameworks offer varying levels of protection. The California Consumer Privacy Act (CCPA), amended by the California Privacy Rights Act (CPRA) in 2023, requires businesses to disclose the use of ADM for profiling in certain contexts, such as targeted advertising. However, unlike the GDPR, the CCPA does not explicitly mandate detailed explanations of the logic or consequences of ADM. Brazil’s Lei Geral de Proteção de Dados (LGPD), effective since 2020, mirrors the GDPR in many respects, including a right to review automated decisions (Article 20), but its enforcement mechanisms are still maturing, with regulatory guidance expected to evolve through 2025.
These frameworks highlight a global trend toward greater transparency, but the depth of disclosure requirements varies, reflecting differing priorities between consumer protection and business interests.
What Information Must Be Provided
Under the GDPR, organizations must provide data subjects with specific information about ADM. First, they must disclose the existence of automated decision-making, including profiling, at the time of data collection (Articles 13 and 14). This ensures individuals are aware that their data may be processed in ways that lead to automated outcomes. Second, they must provide "meaningful information about the logic involved." This does not mean revealing the entire algorithm but rather offering a general understanding of the factors and reasoning behind the decision. For example, in a credit scoring scenario, a bank might explain that the decision was based on income, credit history, and debt-to-income ratio, without disclosing the proprietary weighting of these factors.
Third, organizations must inform data subjects about the "significance and envisaged consequences" of ADM. In the credit scoring example, this might involve explaining that a low score could lead to a loan denial, affecting the individual’s ability to purchase a home. Finally, data subjects must be informed of their rights, including the ability to object to ADM, request human intervention, or contest the decision (Article 22). In a hiring context, a job applicant screened by an algorithm might be told they can request a human recruiter to review their application if rejected.
Practical implementation varies. In credit scoring, companies often provide standardised notices outlining the main factors influencing the score, which satisfies legal requirements while remaining accessible. In contrast, more complex ADM systems, such as those used in predictive policing or healthcare diagnostics, may struggle to distill their logic into meaningful terms without oversimplifying or overcomplicating the explanation.
Challenges in Transparency
Providing meaningful information about ADM is fraught with technical and practical challenges. One major issue is the complexity of modern algorithms, particularly "black-box" models like deep neural networks, where even developers may not fully understand the decision-making process. A 2023 study on explainable AI (XAI) highlighted that while techniques like SHAP (SHapley Additive exPlanations) can identify key features influencing a model’s output, translating these into layperson terms remains difficult. For example, telling a data subject that their loan was denied due to a "non-linear combination of features" is neither meaningful nor helpful.
Another challenge is the protection of trade secrets. Companies argue that disclosing detailed information about their algorithms could compromise competitive advantages. This tension is particularly acute in industries like finance and tech, where proprietary models are a core asset. Additionally, there’s the risk of overwhelming data subjects with technical jargon. A 2024 survey by a privacy advocacy group suggested that over 60% of consumers found existing privacy notices too complex, raising concerns that overly detailed ADM explanations might alienate rather than inform.
Regulatory ambiguity further complicates matters. The GDPR’s requirement for "meaningful information" lacks a precise definition, leaving organizations uncertain about how much detail is sufficient. The EDPB’s 2024 guidelines recommend a case-by-case approach, but without clear benchmarks, compliance remains a gray area, particularly for small organizations lacking resources to develop robust transparency mechanisms.
Balancing Act
The tension between transparency and other interests such as proprietary protection and operational efficiency is a central issue in ADM. On one hand, data subjects have a right to understand decisions that affect their lives, particularly when those decisions involve sensitive areas like employment or access to services. On the other hand, organizations argue that excessive disclosure requirements could stifle innovation or expose them to competitive risks. For instance, a fintech company might hesitate to reveal the logic behind its fraud detection algorithm, fearing that malicious actors could exploit this information.
Regulators and courts have attempted to navigate this balance. The EDPB has clarified that "meaningful information" does not require disclosing the algorithm itself but rather the general principles and criteria used. For example, a 2023 ruling by a German court in a case involving an insurance company upheld that providing a list of factors (e.g., age, claims history) was sufficient, even without detailing their relative weights. However, some advocates argue this standard falls short, particularly for complex systems where the interplay of factors is non-intuitive.
Operational efficiency also plays a role. Providing detailed explanations for every automated decision can be resource-intensive, especially for large-scale systems processing millions of decisions daily. This raises questions about scalability and whether transparency obligations disproportionately burden smaller organizations, potentially creating an uneven playing field.
Best Practices
Organizations can adopt several evidence-based practices to meet transparency obligations while addressing practical constraints. First, they should use layered notices, providing a high-level overview of ADM in initial communications (e.g., "We use automated systems to assess your application based on financial data") with links to more detailed explanations for those who seek them. This approach balances accessibility with depth, catering to varying levels of interest and understanding.
Second, simplified explanations can bridge the gap between technical complexity and meaningful information. For instance, in a hiring algorithm context, a company might explain that the system prioritizes candidates based on years of experience and specific skills, without delving into the model’s mathematical underpinnings. Interactive tools, such as dashboards allowing data subjects to see which factors influenced their decision, can also enhance understanding, as demonstrated by some European banks in 2024.
However, these practices come with trade-offs. Simplified explanations risk omitting critical details, while interactive tools may not be feasible for all organizations due to cost. Additionally, companies must ensure that transparency does not compromise security or competitive interests, which may require collaboration with regulators to define acceptable boundaries.
Contextul Take
Determining how much information needs to be provided to data subjects about automated decisions is a complex issue, shaped by legal, technical, and practical considerations. The GDPR sets a high standard for transparency, requiring meaningful information about the logic, significance, and consequences of ADM, but its implementation remains a subject of debate. Other frameworks, like the CCPA and LGPD, reflect a global push for greater accountability, though their requirements vary in scope and enforcement.
Challenges such as algorithmic complexity, trade secrets, and regulatory ambiguity highlight the difficulty of achieving transparency without overburdening organizations or overwhelming data subjects. As technology evolves particularly with the rise of generative AI and more sophisticated ADM systems these tensions are likely to intensify. Best practices, such as layered notices and simplified explanations, offer a path forward, but they must be continually refined to keep pace with innovation and regulatory developments. Ultimately, the question of transparency in ADM remains an ongoing debate, requiring collaboration among regulators, organizations, and data subjects to ensure a fair balance between efficiency and individual rights.
Comments